Warning
Brace yourselves for an unexpected written journey into the world of statistical theory. This article is about to dive into plenty of nerdy goodness, where numbers take court, algorithms are king, and Bayesian models throw a statistical revolution.
If you were expecting my usual strength and conditioning coaching stories or leadership advice, fear not—it's taking a coffee break while we embark on a statistical adventure.
This post may contain an overdose of statistical humor, Bayesian puns, and the occasional appearance of the elusive Data Goblin. If you're allergic to equations, probability distributions, or overly enthusiastic discussions about priors, consider this your friendly cautionary tale. However, if you find joy in numbers and the artistry of statistical concepts, buckle up, because we're about to take an adventure with data.
Introduction
Bayesian modeling, often overlooked in the realm of Athletics, Coaching, and Strength & Conditioning, holds great promise for optimizing training strategies. It diverges from traditional approaches, offering unique advantages. In this article, I steer away the traditional Bayesian focus from statistical-heavy theory and applied applications within business and refocus the spotlight on the application of Bayesian principles within strength and conditioning coaching for athletes in team sport.
This initial article introduces a hypothetical scenario that often plays out within athletic departments nation-wide; attempting to better individualize workloads and training programs. In this example, I attempt to showcase why Bayesian methodologies are indispensable in tailoring training programs. Unlike conventional methods, Bayesian modeling lets us bypass arbitrary assumptions, providing not just point estimates but entire distributions of potential outcomes.
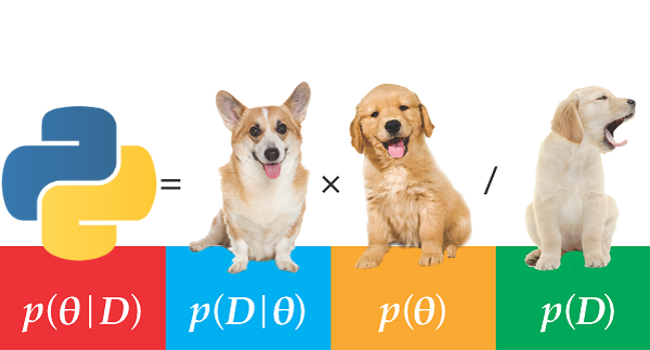
The Athletic Case
Meet the scenario: a collegiate athletics program, working with sports like women's volleyball and basketball. The goal is to optimize training strategies in a Bayesian fashion. This goes beyond merely boosting physical performance; it involves understanding the distinctiveness, sensitivity to training, and uncertainties in each sport.
Understanding the Coaching Challenge
Before proceeding, let's scrutinize potential challenges in past coaching strategies. Analyzing historical training data reveals variations in performance across sports.
Why do athletes in one sport respond differently to training than those in another?
Perhaps, like in our example, athletes in women's basketball exhibit greater responsiveness than those in volleyball due to specific physical demands. One-size-fits-all training strategies may not be effective. By quantifying training sensitivity for each sport, we can tailor coaching strategies more effectively.
On a positive note, having data on both successful and unsuccessful training outcomes for various programs provides a fertile ground for Bayesian analysis. Bayesian approaches not only predict outcomes but also incorporate coach insights into the model. If assumptions about training responses prove inaccurate, the data corrects the course.
Bayesian Principles in Athletic Coaching
In a nutshell, Bayesian modeling employs a variation of Bayes Theorem to unveil the true nature of athletic performance given observed data and existing coaching insights. This contrasts with traditional methods, minimizing the need for questionable assumptions or arbitrary thresholds. Bayesian modeling incorporates prior coaching knowledge, making the process intuitive, transparent, and less prone to misinterpretation.
Bayesian modeling goes beyond point estimates, offering distributions of possible training parameters.
Engineering a Bayesian Coaching Model
In aiding the athletic program, the goal is to apply Bayesian modeling to understand the relationship between training strategies and athletic performance. The focus is on obtaining posterior distributions of training sensitivity parameters.
Likelihood Distribution
The Likelihood Distribution represents the statistical relationship that elucidates how probable observed training data is given the coaching model. This is particularly relevant when working with wearable GPS or accelerometry technologies that capture an athlete's movements and physiological responses.
The Likelihood Distribution, often modeled using Bernoulli distributions in the context of binary training outcomes (such as successful completion of a workout or an occurrence of fatigue), quantifies the probability of specific training outcomes based on the collected data. For instance, strength and conditioning coaches can utilize this distribution to understand how likely an athlete is to achieve optimal performance or experience fatigue under certain workload conditions, offering insights that guide training adjustments and performance optimization strategies.
- Represents the statistical relationship indicating how likely observed training data is given our coaching model.
- Often modeled using Bernoulli distributions, reflecting binary training outcomes.
Prior Distribution
The Prior Distribution in athletics acknowledges the initial coaching knowledge and beliefs. In the Bayesian framework, strength and conditioning coaches can incorporate their domain expertise, experience with specific athletes, and general training principles into the prior distribution. This becomes essential when dealing with the individualized nature of athlete responses to workload. Uninformed priors, chosen when little is known about a particular athlete or when generalizations need to be avoided, allow for a broad range of possibilities.
For instance, a strength coach may have prior beliefs about an athlete's susceptibility to injury, recovery patterns, or optimal training intensities. The Prior Distribution allows coaches to integrate this valuable qualitative knowledge into the quantitative modeling process, resulting in a more personalized and contextually relevant approach to workload management.
- Acknowledges initial coaching knowledge and beliefs.
- Uninformed priors are chosen if little is known, allowing for a broad range of possibilities.
Technical Parameter
The Technical Parameter in the athletics context represents a data-dependent constant value that might be less intuitive but is essential for practical modeling.
This parameter captures the underlying technical aspects of the data that may not be directly interpretable but are crucial for the Bayesian model's functionality. For wearable technologies, this could involve factors such as the precision and accuracy of the data collected, the calibration of the wearable sensors, or the normalization of workload metrics.
Understanding and accounting for these technical parameters are vital to ensure that the Bayesian model accurately reflects the nuances of the data, leading to reliable insights that coaches can trust when making decisions related to athlete training loads and performance optimization.
- Data-dependent constant value, less intuitive but essential for practical modeling.
Bayes Theorem guides the combination of these components into a single equation, shaping the prior distribution into a more informed posterior distribution. Essentially, our initial understanding of training dynamics evolves with new data.
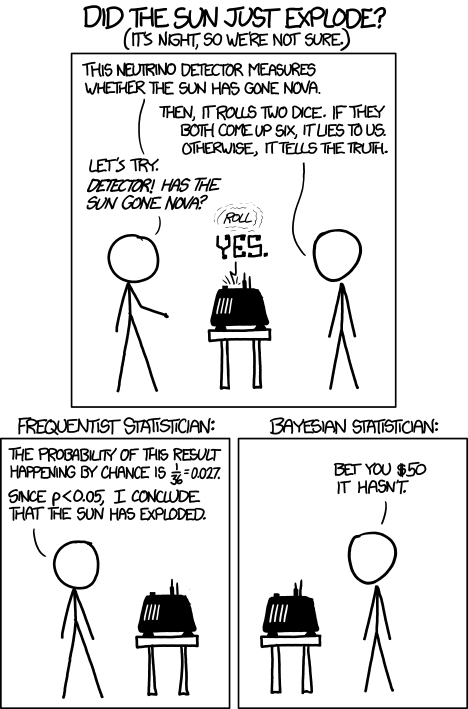
Translating the Athletic Case
To conclude this initial segment, let's translate the coaching challenge into Bayesian terms. The objective is to gain better insights into training behaviors and differences in how sports respond to coaching strategies.
Primarily, we seek posterior distributions for training sensitivity parameters, envisioning distributions rather than point estimates. This enables a nuanced comparison of sports, not just in averages but also in terms of risks and variations.
How do we acquire these posteriors? By utilizing Bayes formula along with the introduced core components.
Priors incorporate existing coaching knowledge directly into the problem. For instance, as training intensity increases, athletes might exhibit varied responses. Uninformed priors allow for a broad range of possibilities when specific knowledge is limited.
Likelihood distribution addresses the process converting training inputs into outcomes, often modeled using Bernoulli distributions. Utilizing this framework, data is seamlessly integrated into the Bayesian model. The next part of this series will delve into constructing models and analyzing results.
Short Retrospective
In my opening segment of our series on Bayesian Athletic Coaching, I identified a core question in coaching and translated it into the language of Bayesian modeling.
I introduced key concepts, emphasizing transparency and the ability to incorporate prior coaching knowledge. While I defer model construction and analysis to the future articles, the advantages of Bayesian modeling, such as correcting assumptions and providing comprehensive insights, are already evident. This structural approach aids in understanding the essence of coaching challenges, offering not just point estimates but comprehensive information crucial for athletic performance and team success.
Nate Silver, from "The Signal and the Noise"
"Prediction is difficult for us for the same reason that it is so important: it is where objective and subjective reality intersect. Distinguishing the signal from the noise requires both scientific knowledge and self-knowledge: the serenity to accept the things we cannot predict, the courage to predict the things we can, and the wisdom to know the difference”
Bayesian Insights in Athletic Coaching
In the second part of my exploration into Bayesian methodologies for athletic coaching, I delve into the practical applications and benefits for coaches in decision-making frameworks. This approach not only enhances forecasting accuracy but also provides in-depth analytical information for informed decision-making.
Understanding Athlete Responses to Training
In the strength and conditioning coaching profession, understanding how athletes respond to training is crucial for optimizing performance. Bayesian modeling offers a unique advantage by providing a nuanced understanding of training behaviors and variations across different sports and athletes. Instead of relying on traditional approaches that may involve arbitrary assumptions and thresholds, Bayesian statistics allows coaches to incorporate their domain knowledge directly into the model.
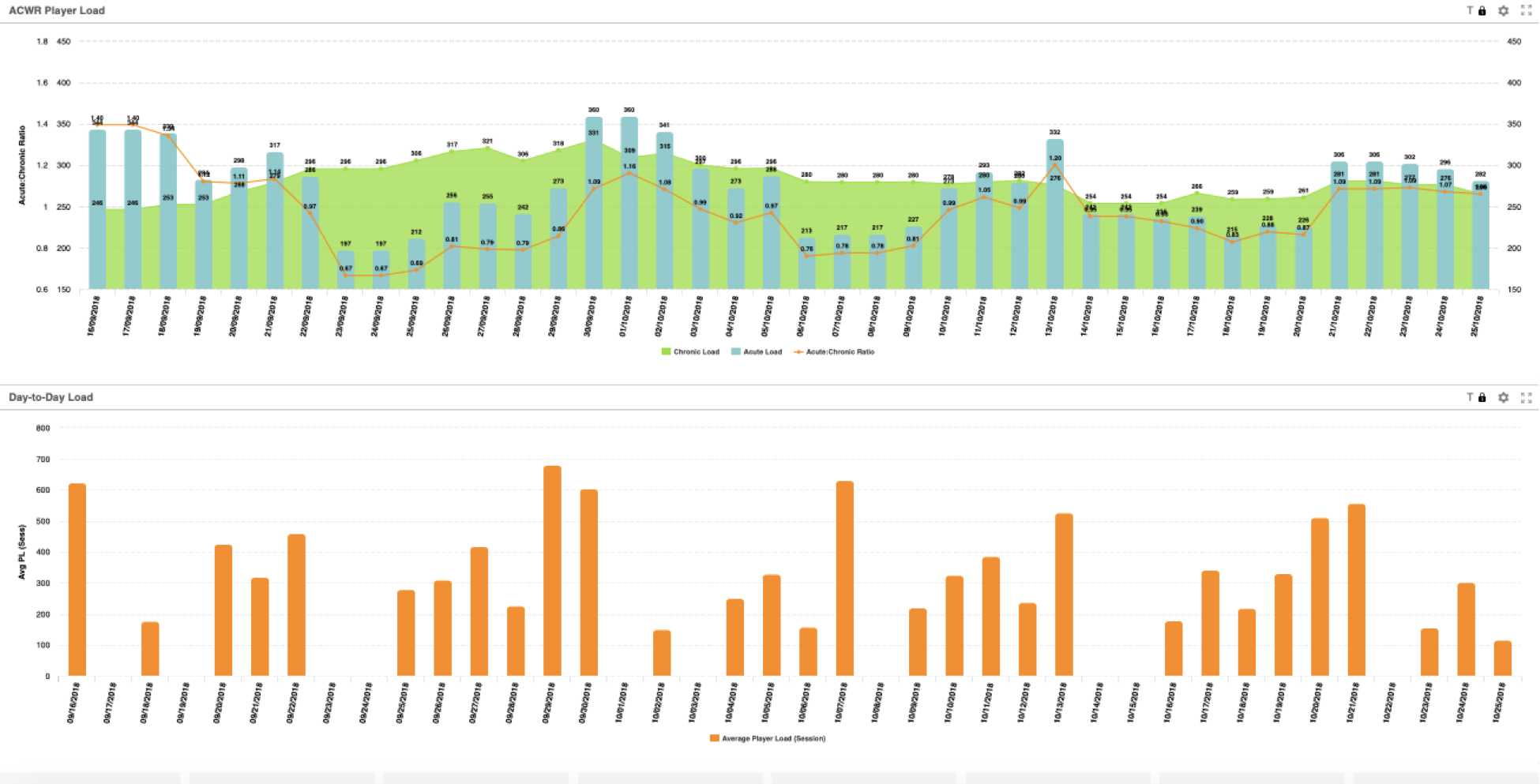
Leveraging Bayesian modeling with wearable GPS or accelerometry technologies such as Catapult Sports or Kinexon Sports can alter the way coaches interpret and utilize workload data for performance optimization. These wearable technologies provide strength coaches with intricate insights into athletes' movements, exertion levels, and physiological responses during training and competition. However, the challenge lies in transforming this data into actionable strategies that enhance athlete performance while mitigating the risk of injuries.
Bayesian modeling offers a solution by allowing coaches to extract nuanced patterns and trends from the complex datasets generated by wearable technologies. Instead of relying solely on deterministic predictions, strength and conditioning professionals can incorporate their domain expertise and prior knowledge into the modeling process. For instance, a strength coach or sport scientist could integrate information about an athlete's injury history, post-game recovery patterns e.g. force plate data and return-to-baseline timelines, and individual response to varying workloads. Bayesian models, particularly in a hierarchical framework, enable the coach to simultaneously consider the unique characteristics of each athlete and identify overarching trends within the team or across different training sessions.
With Bayesian insights, strength and conditioning coaches can make informed decisions about workload management that go beyond traditional approaches. The models provide an ever-changing dynamic understanding of how athletes respond to specific training loads, helping coaches individualize their strength and conditioning programs or loading schemes to fit individual needs. Additionally, Bayesian modeling excels at quantifying uncertainty, allowing coaches to factor in the inherent variability in athletes' responses and adjust training strategies accordingly. This holistic approach enhances the coach's ability to optimize performance, reduce the likelihood of injuries, and foster a data-informed team culture within the coaching staff and athletic team.
Tailoring Training Strategies to Individual Athletes
One key aspect of athletic coaching is tailoring training strategies to individual athletes. Traditional models might struggle to account for the diverse responses seen in different individuals. Bayesian modeling, particularly in a hierarchical framework, acknowledges both differences and commonalities. Strength and Conditioning coaches can gain valuable insights into how athletes from various backgrounds respond to training stimuli.
Dealing with Uncertainty in Decision-Making
In the unpredictable world of athletics, uncertainty is a constant factor. We all know this to be true. Bayesian statistics excels in quantifying uncertainty, providing coaches with a more realistic understanding of the complexities involved. By incorporating prior knowledge and beliefs, coaches can make decisions that are not only data-driven but also account for the inherent variability in athletic performance.
Optimizing Training Strategies Across Teams
For strength coaches overseeing multiple teams or athletes, Bayesian modeling becomes a powerful tool. It allows for the optimization of training strategies across different groups by recognizing similarities and differences in their responses to various interventions. This flexibility enables coaches to make informed decisions tailored to the specific needs of each team or athlete.
Enhancing Decision-Making Frameworks
Bayesian insights enhance decision-making frameworks by offering transparency and a systematic approach. Coaches can confidently integrate their experiential knowledge into the modeling process, ensuring that the model aligns with their understanding of the sport and the athletes they train. The resulting Bayesian model not only improves forecasting accuracy but also provides actionable insights for refining coaching strategies.
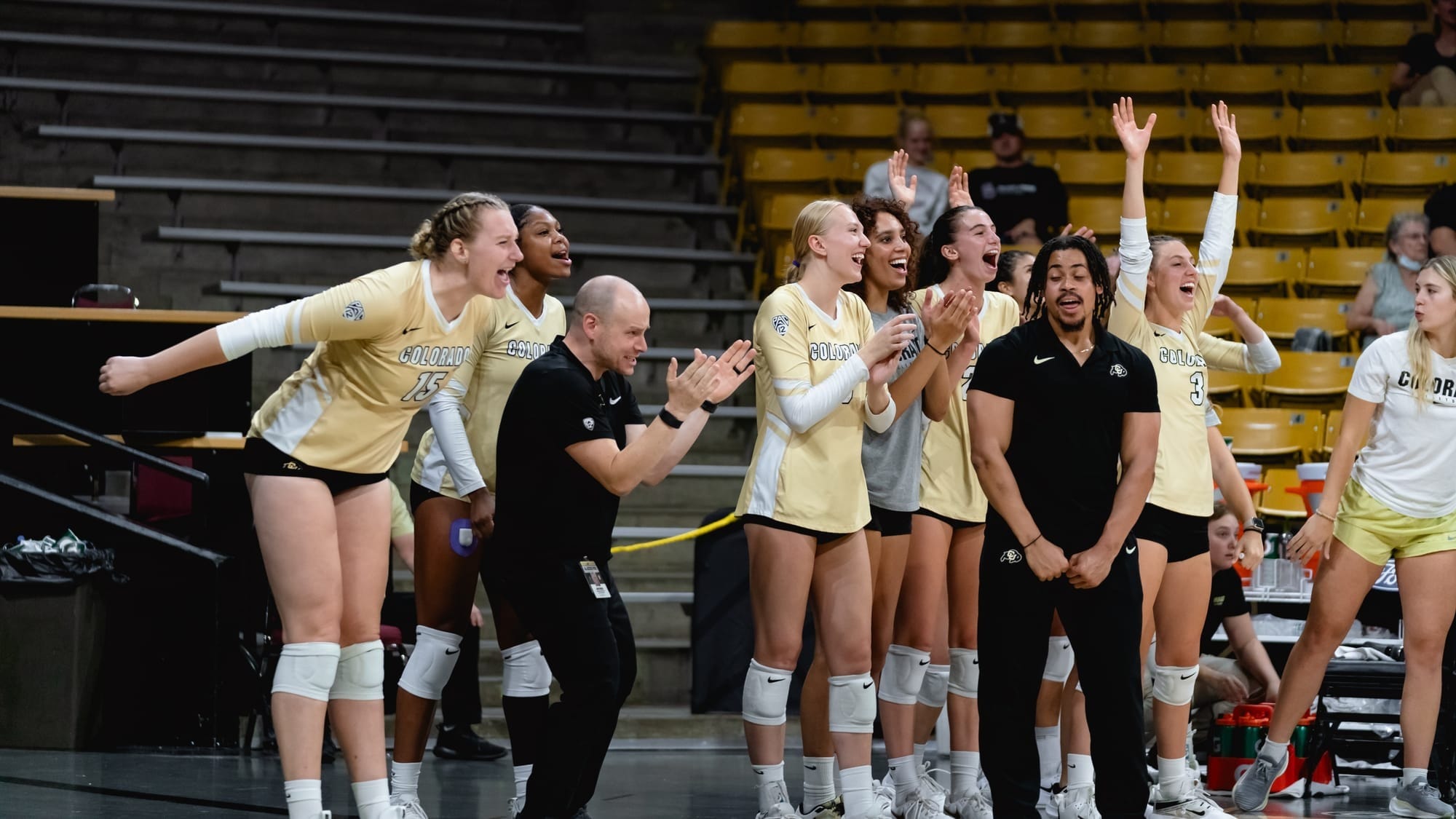
Conclusion
In conclusion, Bayesian statistics is not just a theoretical concept; it is a practical and valuable tool for coaches seeking to optimize training programs. By leveraging Bayesian insights, strength and conditioning coaches can personalize their approaches to individual athletes or group dynamics, better navigate uncertainty, and make informed decisions that lead to improved performance outcomes. The Bayesian framework, with its emphasis on transparency and flexibility, aligns seamlessly with the dynamic and multifaceted nature of athletic coaching.